The Ethics of AI: Building Responsible Systems for Business
Dr. Amelia Rodriguez
AI Ethics Advisor
The Ethics of AI: Building Responsible Systems for Business
As artificial intelligence becomes increasingly integrated into business operations, organizations must consider not just what AI can do, but what it should do. This article explores the ethical dimensions of AI implementation and provides a framework for building responsible AI systems.
Why AI Ethics Matter in Business
Ethical considerations in AI aren't merely academic—they directly impact business outcomes:
Trust and Reputation
Customers increasingly consider a company's ethical stance when making purchasing decisions. AI systems that demonstrate fairness and transparency build trust; those that don't can severely damage reputation.
Regulatory Compliance
Governments worldwide are developing AI regulations focused on fairness, accountability, and transparency. Proactively addressing ethical concerns helps future-proof against regulatory changes.
Risk Mitigation
Unethical AI can create significant legal and financial liabilities through discrimination, privacy violations, or safety failures.
Talent Attraction
Top AI talent increasingly considers ethical practices when choosing employers, making strong AI ethics a competitive advantage in recruitment.
Organizations that lead in ethical AI position themselves for sustainable success in an AI-driven future.
Core Principles of Ethical AI
Responsible AI systems are built on several foundational principles:
Fairness and Non-discrimination
AI systems should provide consistent and unbiased results across different demographic groups and user segments. This requires careful data selection, algorithmic design, and ongoing monitoring for unintended bias.
Transparency and Explainability
Users should understand how AI systems make decisions, particularly for high-stakes applications. This may require interpretable models, confidence scores, or explanations for specific recommendations.
Privacy and Security
AI systems should protect sensitive data through anonymization, encryption, access controls, and secure development practices. User data should only be used in ways that align with reasonable expectations.
Human Oversight and Control
AI should augment human decision-making rather than replace it entirely, especially for consequential decisions. Clear mechanisms should exist for humans to review, override, or appeal automated decisions.
Accountability
Organizations must take responsibility for their AI systems' impacts, establishing clear ownership, governance structures, and remediation processes for when systems cause harm.
These principles provide a foundation for ethical AI development and deployment.
Implementing Ethical AI: Practical Approaches
Moving from principles to practice requires concrete implementation strategies:
Diverse Development Teams
Include people with varied backgrounds, experiences, and perspectives in AI development to identify potential blind spots and biases early.
Ethical Risk Assessment
Before development begins, conduct a thorough assessment of potential ethical risks and develop mitigation strategies for each identified risk.
Representative Data
Ensure training data represents the full diversity of users and use cases, with particular attention to historically underrepresented groups.
Regular Bias Testing
Implement testing regimes to detect bias in both training data and model outputs, with specific metrics for fairness across different user segments.
Explainability Methods
Develop appropriate explanation methods based on use case criticality, from simple confidence scores to detailed rationales for specific decisions.
Ongoing Monitoring
Establish systems to continuously monitor AI performance in production, watching for unexpected behaviors, emergent biases, or changing societal standards.
Clear Documentation
Document design choices, limitations, intended uses, and ethical considerations throughout the development process for both internal and external stakeholders.
User Feedback Channels
Create accessible mechanisms for users to report concerns, request explanations, or appeal decisions made by AI systems.
These practical approaches help translate ethical principles into operational reality.
Governance Frameworks for Ethical AI
Sustaining ethical practices requires formal governance structures:
Ethics Committees
Establish cross-functional committees including technical, legal, business, and ethics experts to review high-risk AI initiatives.
Ethical Guidelines
Develop clear, specific guidelines for AI development that operationalize ethical principles for your organization's context.
Training Programs
Implement ethics training for all staff involved in AI development, from data scientists to product managers and executives.
Third-Party Reviews
Consider independent audits or reviews of high-stakes AI systems before deployment.
Impact Assessments
Conduct formal impact assessments for AI systems with significant potential effects on individuals or society.
Effective governance ensures ethical considerations are systematically addressed rather than treated as afterthoughts.
Conclusion
Building ethical AI systems isn't just the right thing to do—it's a business imperative. Organizations that embed ethical considerations into their AI development processes protect themselves from reputational damage and regulatory challenges while building sustainable competitive advantage.
As AI becomes more powerful and pervasive, the organizations that lead in responsible implementation will earn the trust needed to fully realize AI's transformative potential. The choice is clear: ethical AI is not a constraint on innovation but the only sustainable path forward.
Dr. Amelia Rodriguez
AI Ethics Advisor
Dr. Amelia Rodriguez is a contributor at Buildberg, sharing insights on technology and business transformation.
Related Posts
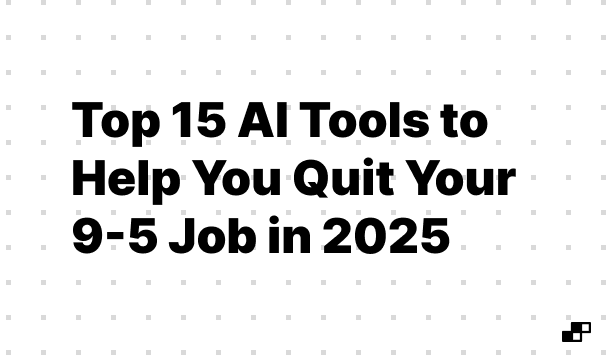
Top 15 AI Tools to Help You Quit Your 9-5 Job in 2025
Discover the most powerful AI tools that can help you build a sustainable online business and leave your traditional job behind.
Read more