Reasoning Agents: How to Build Powerful AI Agents with Chain of Thought Capabilities
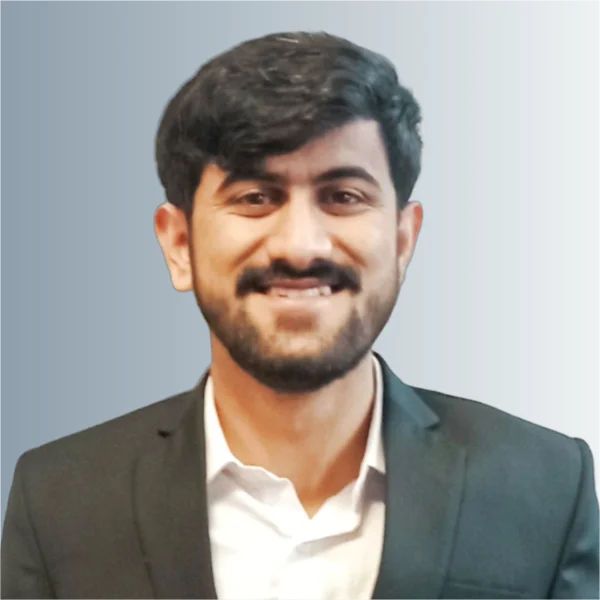
Usama Navid
AI and Automation Expert
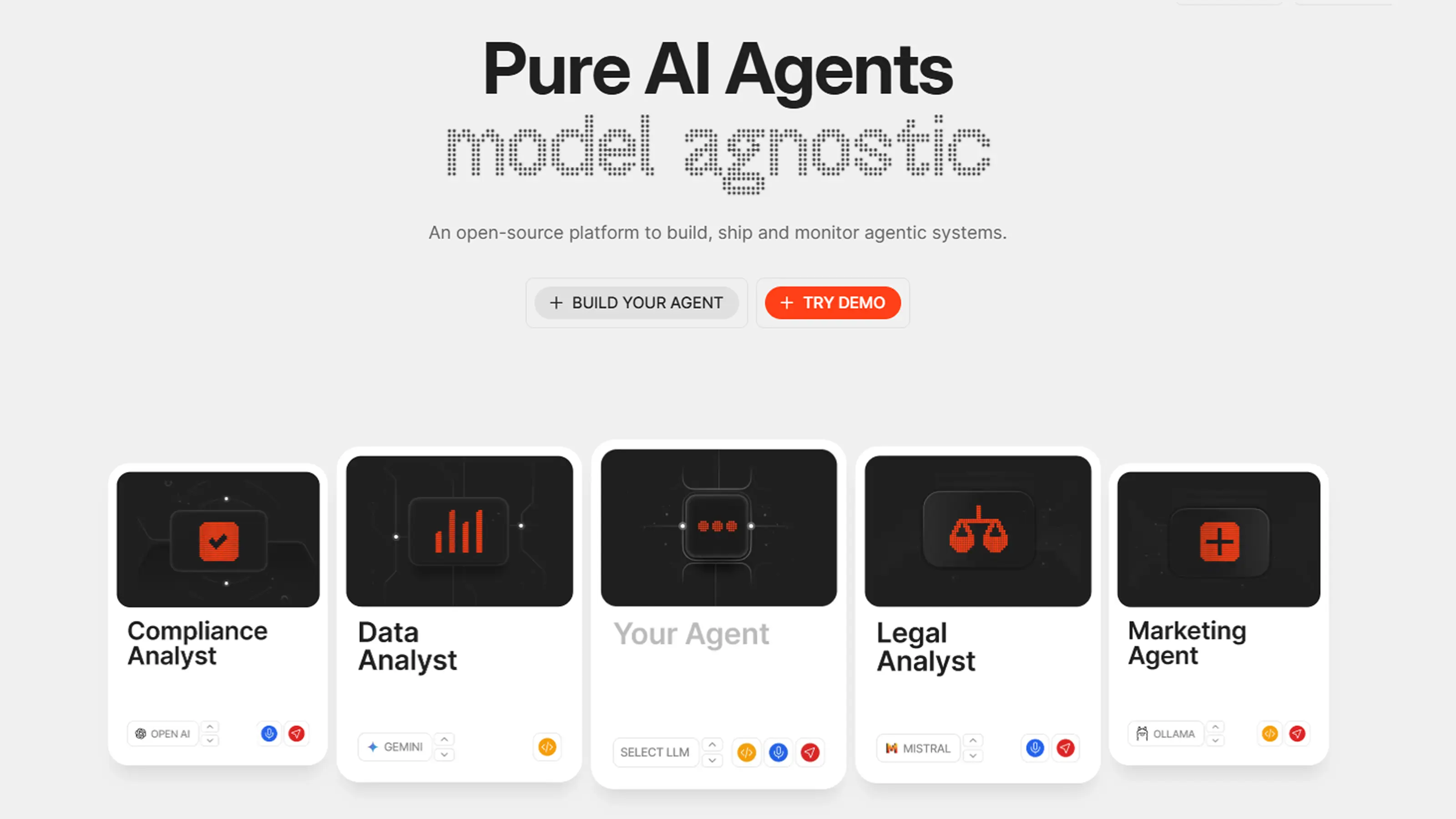
What Are Reasoning Agents?
Reasoning Agents represent a significant advancement in multi-agent AI systems. Developed by Agno, these agents combine chain-of-thought reasoning with tool utilization to solve complex problems more effectively. The most remarkable aspect? You can transform virtually any AI agent into a reasoning agent with minimal code changes.
As highlighted in recent developments, reasoning capabilities aren't limited to massive models like GPT-4 or Claude. Even smaller, locally-runnable models can demonstrate impressive reasoning when properly configured.
How Reasoning Agents Work
When an agent with reasoning enabled receives a task, it follows a sophisticated process:
- A dedicated "Reasoning Agent" first approaches the problem using chain-of-thought methodology
- At each step, it calls appropriate tools to gather information and validate results
- The agent iterates through the reasoning process until reaching a conclusion
- Finally, it returns the results to the original agent for validation and response formulation
What makes this approach powerful is the combination of methodical thinking with tool access, enabling even smaller models to tackle complex problems.
Setting Up Your First Reasoning Agent
Creating a reasoning agent requires just a few lines of Python code using the Agno library:
The magic happens with just one parameter: reasoning=True
. This simple addition transforms a standard agent into one capable of breaking down problems systematically.
Running Reasoning Agents Locally
One of the most exciting aspects demonstrated in recent tutorials is the ability to run reasoning agents locally using smaller models. For example, you can use Ollama with models as small as 1.5 billion parameters:
This approach democratizes access to advanced AI reasoning, making it available even on machines with modest specifications (8-16GB RAM).
Use Cases for Reasoning Agents
The applications for reasoning agents are diverse and powerful:
1. Creative Writing
Even smaller models can generate impressive creative content when equipped with reasoning capabilities. In tests, a 1.5 billion parameter model successfully created a short story about life in 5 million years by:
- Breaking down the problem scope
- Identifying different storytelling approaches
- Considering interdisciplinary perspectives
- Building a cohesive narrative framework
2. Code Explanation
Reasoning agents excel at explaining complex code. For example, when presented with Python list comprehension code:
A reasoning agent breaks down the logic step by step, making it accessible even to beginners.
3. Financial Analysis
With appropriate tools, reasoning agents can analyze financial data comprehensively:
4. Logical Puzzles and Mathematical Proofs
Reasoning agents can tackle classic problems like the missionaries and cannibals puzzle or mathematical proofs with structured approaches.
5. Ethical Analysis
Complex ethical dilemmas benefit from reasoning agents' ability to consider multiple frameworks and perspectives simultaneously.
Requirements for Reasoning Models
To implement reasoning capabilities:
- Use a model that supports structured outputs (like GPT-4o, Claude 3.7 Sonnet, or Gemini with JSON mode)
- Alternatively, use native reasoning models like o3-mini or deepseek-r1
- Ensure your model has access to appropriate tools for the task at hand
Why This Matters for AI Development
Reasoning agents represent a significant step forward in making AI more useful and accessible:
- Democratization: Even smaller, locally-runnable models can tackle complex problems
- Transparency: The reasoning process is visible, making AI decision-making more interpretable
- Versatility: The same approach works across different model sizes and types
- Complexity management: Breaking problems into steps helps models handle nuanced tasks
Getting Started with Agno
Agno is a completely open-source library, meaning you can start building reasoning agents without any cost. The framework provides:
- Simple integration with various models (OpenAI, Claude, local models via Ollama)
- Built-in tools for various domains
- The ability to create custom tools
- Comprehensive documentation and examples
Conclusion
Reasoning Agents demonstrate that AI's value comes not just from raw model size but from how we structure problem-solving approaches. By enabling chain-of-thought reasoning and tool use, even smaller models can tackle complex tasks with surprising effectiveness.
Whether you're looking to build locally-running agents or enhance cloud-based solutions, the reasoning agent approach offers a powerful paradigm for more capable AI assistants.
Ready to build your own reasoning agent? Check out the Agno documentation and explore the examples in their Reasoning Agents Cookbook.
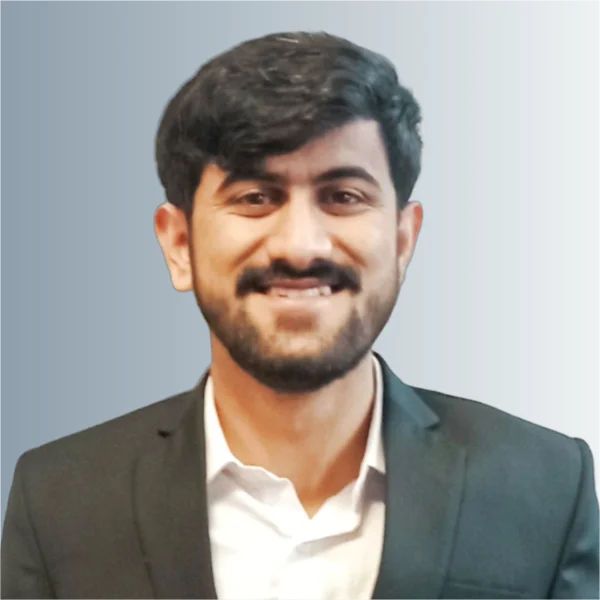
Usama Navid
AI and Automation Expert
Usama Navid is a contributor at Buildberg, sharing insights on technology and business transformation.
Related Posts
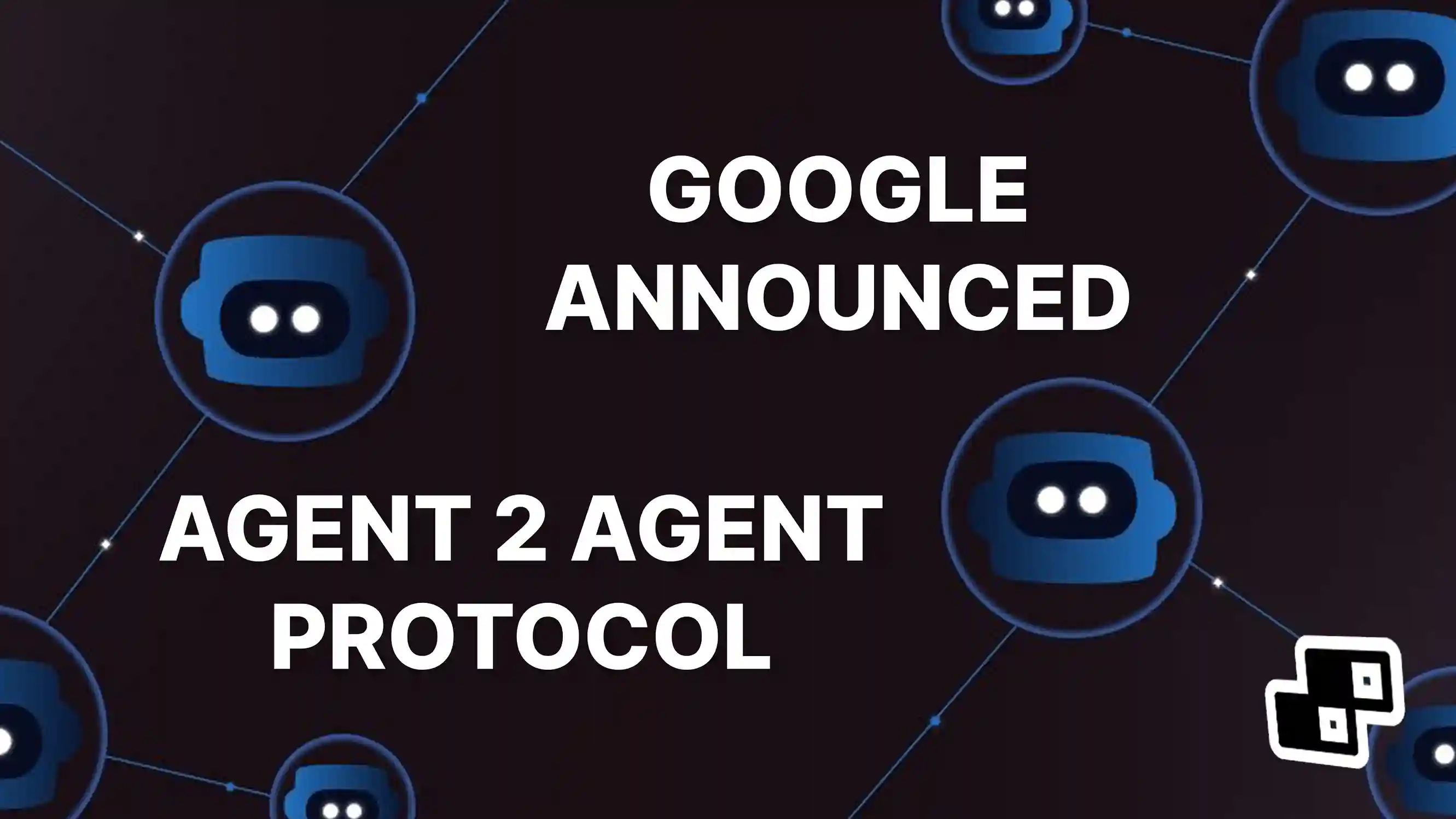
Agent2Agent Protocol: Google's New Framework for AI Collaboration
Google Cloud Next 2025 introduced the Agent2Agent (A2A) Protocol, a new open standard designed to revolutionize how different AI agents communicate and collaborate, regardless of their underlying models or frameworks.
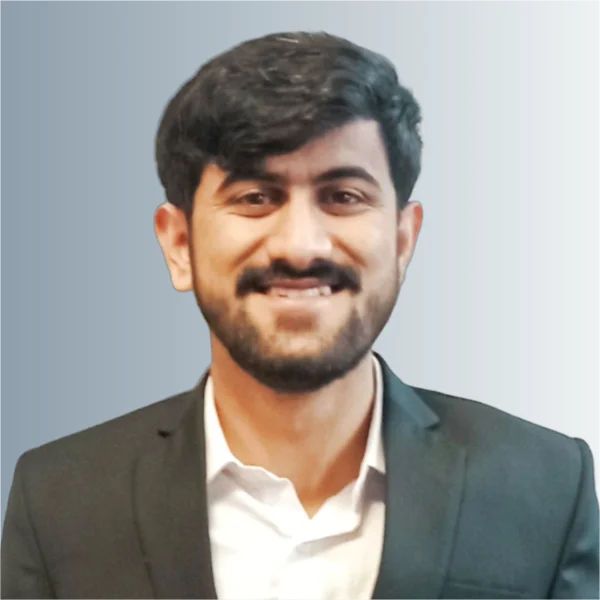
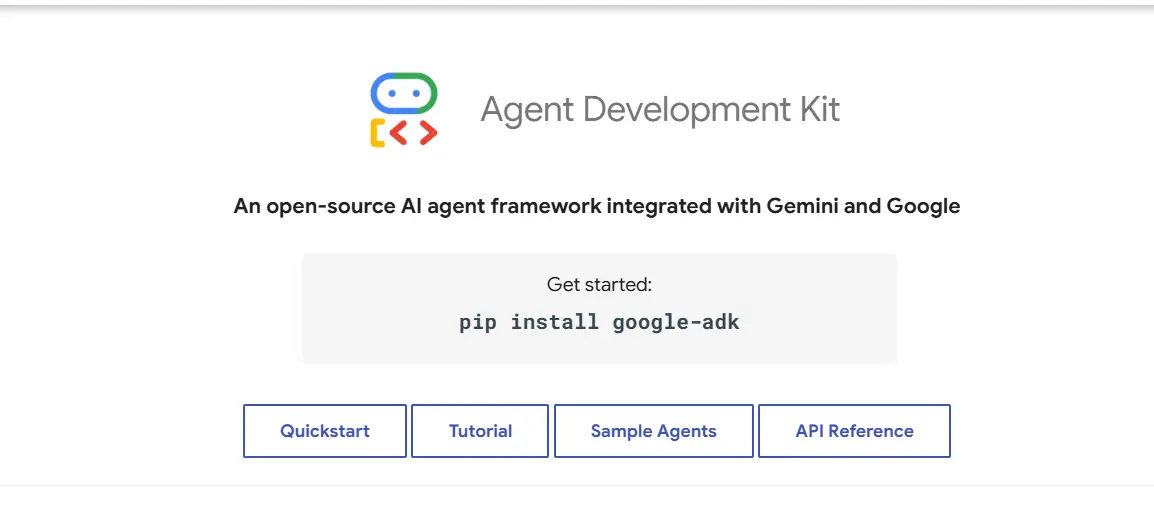
Google's Agent Development Kit (ADK): A New Era for AI Agent Development
Google unveils its Agent Development Kit (ADK), a Python-based toolkit designed to revolutionize how developers build, evaluate, and deploy AI agents with seamless cloud integration.
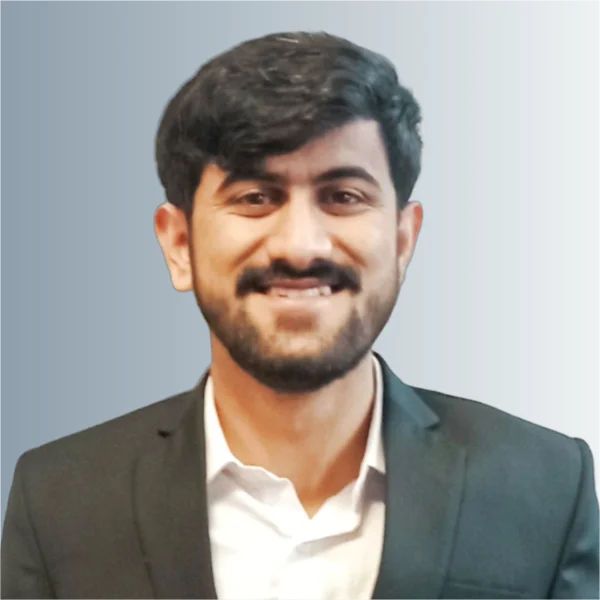